Annika Hauser & Florian Stahl
If marketing or sales managers want to create customer experiences that go beyond just delivering the right product to their customers, they can’t avoid dealing with one very important technology – artificial intelligence (or short AI). This article aims at illustrating how different applications of AI can be deployed by companies in order to fulfill customers’ experiential needs that arise along the customer journey.
In this day and age, consumers no longer want a product or service to simply fulfill its basic functionality, like e.g., being able to get from A to B when purchasing a car.[1] In virtually every industry, product features are becoming easier to imitate by other brands, which makes it harder for companies to differentiate themselves through their products alone. Clearly, companies must seek a competitive edge elsewhere – and this is where superior customer experience comes into play.[2] Take Tesla, for example: Through AI, Tesla monitors everything that is going on in their cars. In doing so, Tesla is able to predict issues like part failures before the customer even knows that something is wrong. As a result, Tesla can immediately provide the necessary service to the customer upon occurrence of the actual failure, thereby ensuring a superior customer experience.[3]
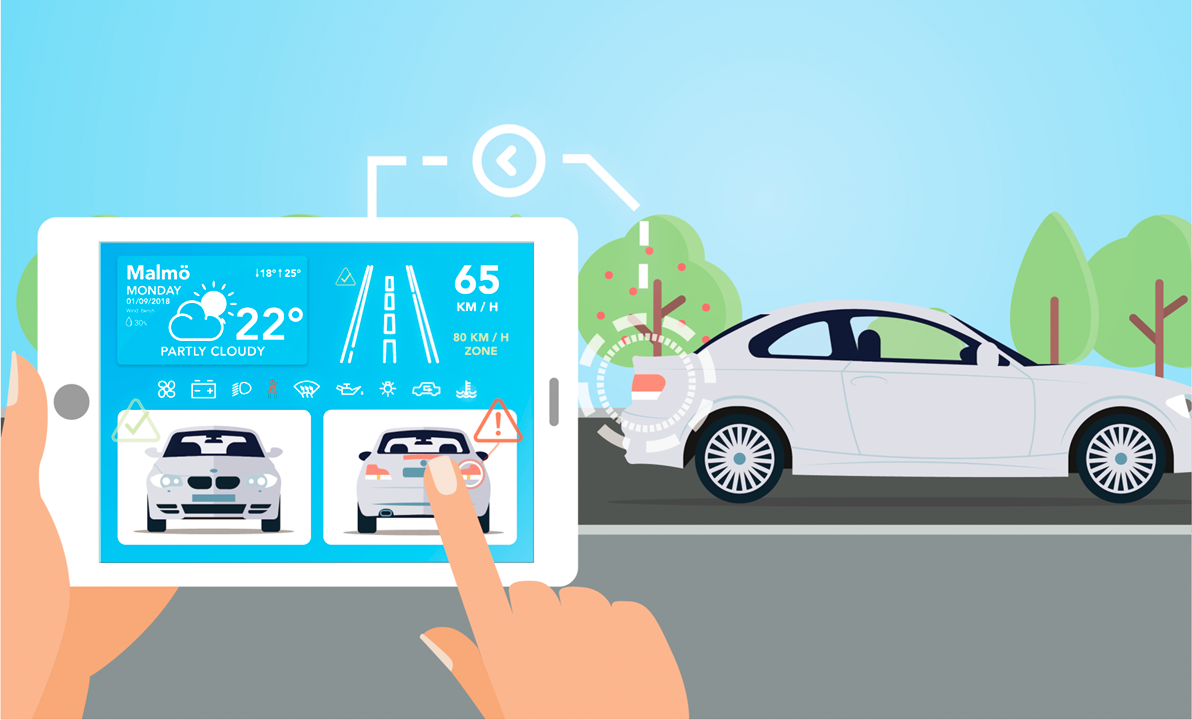
Basically, customer experiences emerge every time value is transferred from a seller to a buyer. This includes all parts of the customer journey, meaning that those experiences do not only occur when a person buys a product, but also when a person consumes a product or service and even when a person searches for a product or service online before the actual purchase even happened.[4][5]

Due to the digital revolution, we observe tremendous change regarding the concept of customer experience. When customer experience first became a relevant concept to the disciplines of marketing and sales, customers were viewed as passive entities who just responded to companies’ offerings. Today, in times of AI, the Internet of Things, and smart devices, customers actually interact with such devices, which brings whole new considerations to the concept of customer experience.[6] Digital advancements further enable companies to add experiences to virtually all touchpoints with their customers. Coca Cola, e.g., uses AI in China to bring interactivity to the purchase of drinks from vending machines: when customers buy a coke, they, for instance, receive a message on their smartphone containing a reminder to recycle the bottle. Recycled bottles then result in credits that can be shared with friends or spent on new purchases.[7]
To get a better grasp on how different AI tools can deliver superior customer experiences, one should be familiar with the underlying customer needs; whether those needs are satisfied or not, determines if the customer evaluates an experience as positive or negative.
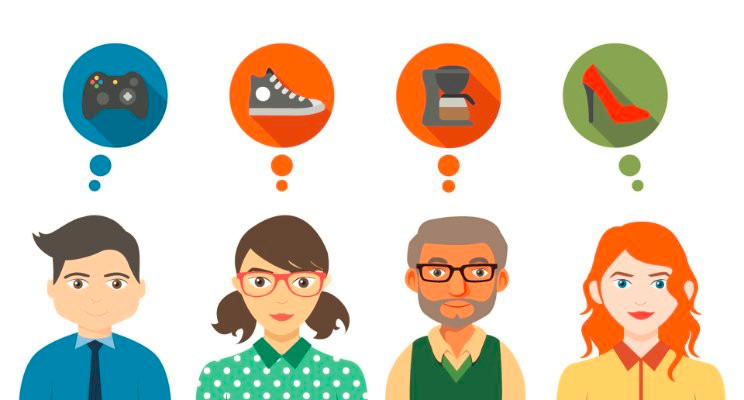
First, because the digital revolution brings along countless possibilities for product and service design, customers increasingly desire personalized experiences that match their personality.[8] This is especially relevant, as offerings from different companies become less differentiated: if customers cannot identify any other differences between products or services, they will choose the one that best matches their personality.[9]
Second, driven by technological advancements, customers seek experiences that satisfy a need for meaningful interactions. As mentioned before, in a world in which customers are connected to firms, products, or other customers through technology, they are no longer silent observers – instead, customers can and want to interact with other actors and actively participate in the value creation process.[10]
Third, another experiential customer need driven by technology is the need for immediacy. Today, it is easy for customers to access products and services – but also information about products and services – quickly through diverse channels. Due to this development, customer expectations have risen. This means that whenever customers have a question, they demand a prompt answer, or else they might just move on to another product or service.[11]
Fourth, customers naturally seek to increase their overall well-being through avoiding decision regret. Customers are confronted with endless, often overwhelming information streams, which increases the risk of fearing that a better consumption decision could have been made. Such decision regret will lower customers’ well-being and result in a negative customer experience.[12]
Which AI tools are relevant for creating superior customer experiences?
To understand how AI can be leveraged for superior customer experiences, marketing and sales managers need to be able to distinguish between different AI tools. But before diving into specific applications, let’s first define what AI actually is: the term artificial intelligence refers to machines, systems, algorithms, or programs that are capable of performing intellectual tasks that would otherwise be performed by humans.[13] Machine learning, natural language processing, and neural networks are some key technologies that enable AI to perform those intellectual tasks[14] and collect and analyze data to learn from the insights. Based on these insights, AI can continuously and flexibly adapt.[15]
In a marketing context, AI allows companies to solve diverse marketing problems by making predictions based on historical customer data. Hereby, AI has proven to be faster and more accurate than humans in making predictions.[16]
Next, we will have a closer look at distinct forms of applying AI in business contexts.

Firstly, companies deploy AI to personalize products or services, for instance through recommender systems. Such recommender systems serve customers with recommendations that are based on their personal behavior. Here, the AI-application is based on machine learning algorithms that identify patterns from customer data.[17] Many best-in-class examples of companies exist that successfully harness recommender systems to cater to their customers. Take Netflix or Amazon, for example: based on customers’ previous behavior, the firms successfully make recommendations to customers about what to watch or buy.[18]
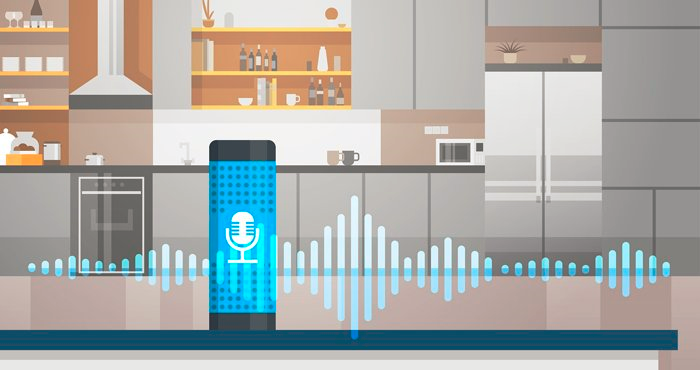
Secondly, AI can be deployed in the form of (voice-controlled) smart agents. Such AI-driven smart devices can interact with customers or other smart devices through the internet and make autonomous decisions to adjust to context-related circumstances.[19] Like recommender systems, those agents rely on pattern recognition through machine learning algorithms. They further use natural language processing technologies to be able to interact with customers via voice or text.[20] One very popular voice-controlled smart agent is Amazon’s Alexa, which is able to perform different tasks, like playing a Spotify playlist, upon the user’s verbal request.[21]
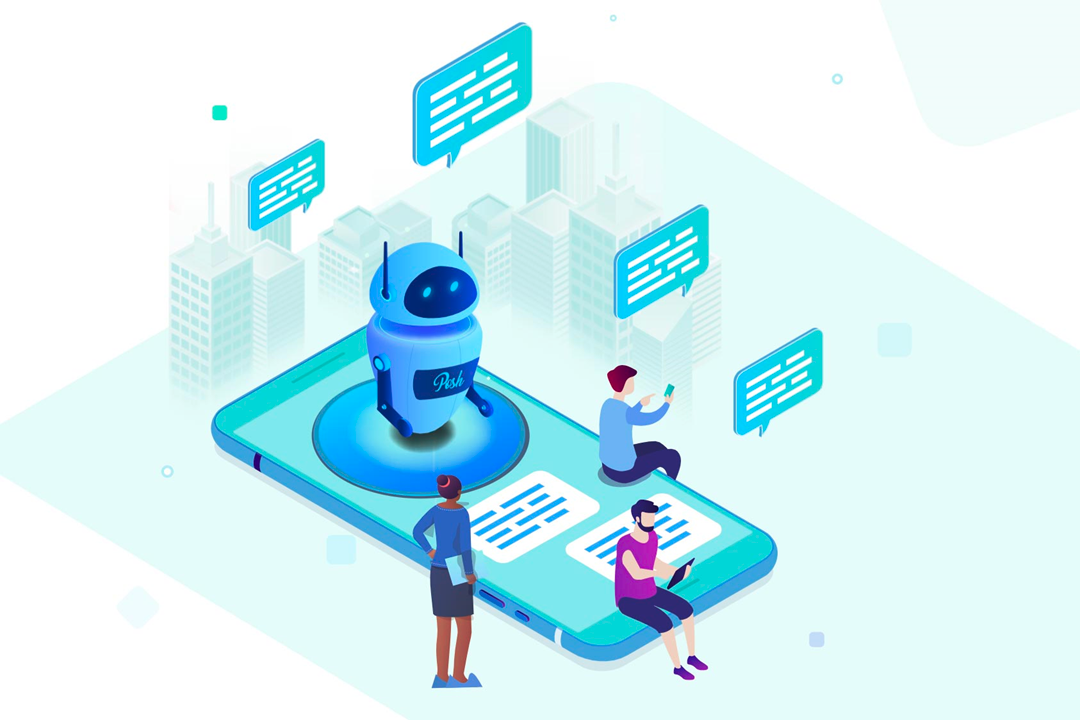
Thirdly, in order to improve customers’ service experiences, many companies use AI in the form of chatbots. Chatbots imitate human conversations in order to interact with customers. Hereby, the conversation can take place via a textual chat or via a verbal phone call.[22] Regarding the technology, chatbots function in a manner comparable to (voice-controlled) smart agents. However, the use of chatbots is usually limited to website visits and service calls.[23]
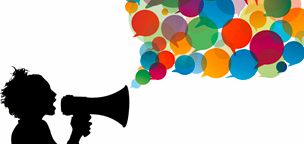
Fourth, especially for marketing purposes, AI can be used in the form of supervised machine learning techniques for the analysis of textual and other contextual data that are able to detect response-worthy electronic word-of-mouth on social media. Such machine learning algorithms are trained on data that is pre-classified as response-worthy or not. They then learn to predict whether large amounts of unclassified data are response-worthy. These techniques capture the context in which a social media post or comment was written and how relevant the content is to the business. This enables companies to identify relevant comments or posts and, in turn, create meaningful interactions with customers.[24]
How can the different AI applications satisfy customers’ experiential needs?
The following table visualizes which AI application fulfills which experiential customer need:
As shown in the table, recommender systems have the potential to meet the experiential needs of personalization, well-being (avoidance of decision regret), and – to some extent – interaction. It is quite clear how recommender systems fulfill a customer’s need for personalization: The machine learning algorithms powering recommender systems learn customers’ preferences and then adapt to them to recommend products that best suit each individual customer. Customers, in turn, have a better experience, because they feel like the company that makes appropriate recommendations knows and understands them.[25] Further, recommender systems address customers’ need for increased well-being through avoiding decision regret.[26]
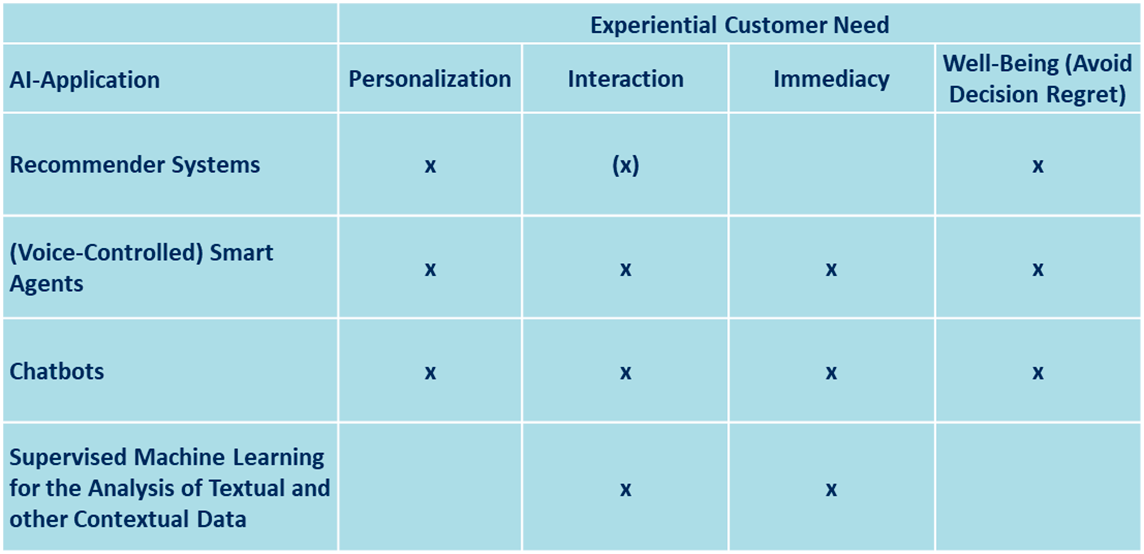
The information flood customers are confronted with in most digital contexts can cause cognitive stress as customers seek to make the best possible choice regarding, for example, a product to purchase or a movie to watch. When confronted with such a vast number of choices, customers often fear regretting their decision later on, which can cause serious distress or might even force customers to abandon the decision process. Here, recommender systems come in play and narrow down customers’ choices. This greatly improves the customer experience, because it allows customers to process all the information and make a decision with greater confidence and less fear of decision regret. Lastly, if recommendations are framed in a way that makes customers feel like the recommendation was made by another customer (e.g., by recommending products that were purchased by other consumers with similar preferences), recommender systems can give customers a sense of interaction. However, this effect is limited, because no “real” interaction takes place.[27]

The next AI-powered tools – (voice-controlled) smart agents – are able to create superior customer experiences by fulfilling all four of the introduced experiential customer needs. Regarding personalization, smart agents exhibit an enormous potential: smart agents can adapt to customers’ behaviors and habits in a way that allows for a strong bond between the device and the customer. This strong bond can result in a trusting relationship between the agent and the customer and, thus, in a greatly improved customer experience.[28]
Further, (voice-controlled) smart agents are a very powerful tool when it comes to fulfilling customers’ need for interaction. Imagine, for instance, a customer’s verbal interaction with Amazon’s Alexa. During the interaction, the customer perceives Alexa to behave – to some extent – in a human manner. This makes the interaction enjoyable and allows the customer to add an interaction component to many daily routines like listening to music by asking Alexa to play a playlist.[29] Customers’ need for immediacy can also be effectively addressed by smart agents. As they are powered by AI, smart agents can react to customer requests in real-time and from any location or during any time of the day.[30] The need for increased well-being can be addressed by (voice-controlled) smart agents analogously to recommender systems. As smart agents adapt to customers’ preferences and behaviors, they can provide customers with tailored recommendations upon request, which will – due to the trusting relationship that often exists between customers and (voice-controlled) smart agents – lower the risk of decision regret.[31]
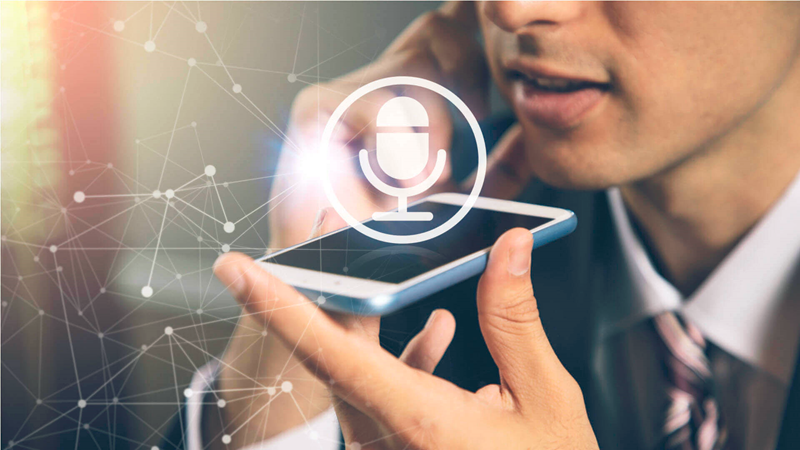
Like (voice-controlled) smart agents, chatbots are able to fulfill all four experiential customer needs. As mentioned before, chatbots exhibit traits comparable to smart agents, while being mostly limited to website visits and service calls.[32] Regarding personalization, the AI that powers chatbots is able to fully adapt to customers’ needs when they interact with the chatbot during a website visit or service call. Chatbots are also able to meet customers’ need for interaction, because they add a human-like interaction component to activities like online shopping, when providing the customer with support. Such activities would otherwise not include any form of interaction, which leads to an improved customer experience.[33] When it comes to satisfying customers’ need for immediacy, chatbots are particularly effective: the AI powering chatbots is constantly available, allowing to serve customers with immediate personalized answers or solutions.[34] Lastly, chatbots can help avoid decision regret in the same way as (voice-controlled) smart agents can, namely, by providing customers with appropriate product or service recommendations that are based on a customer’s preferences upon request.[35]
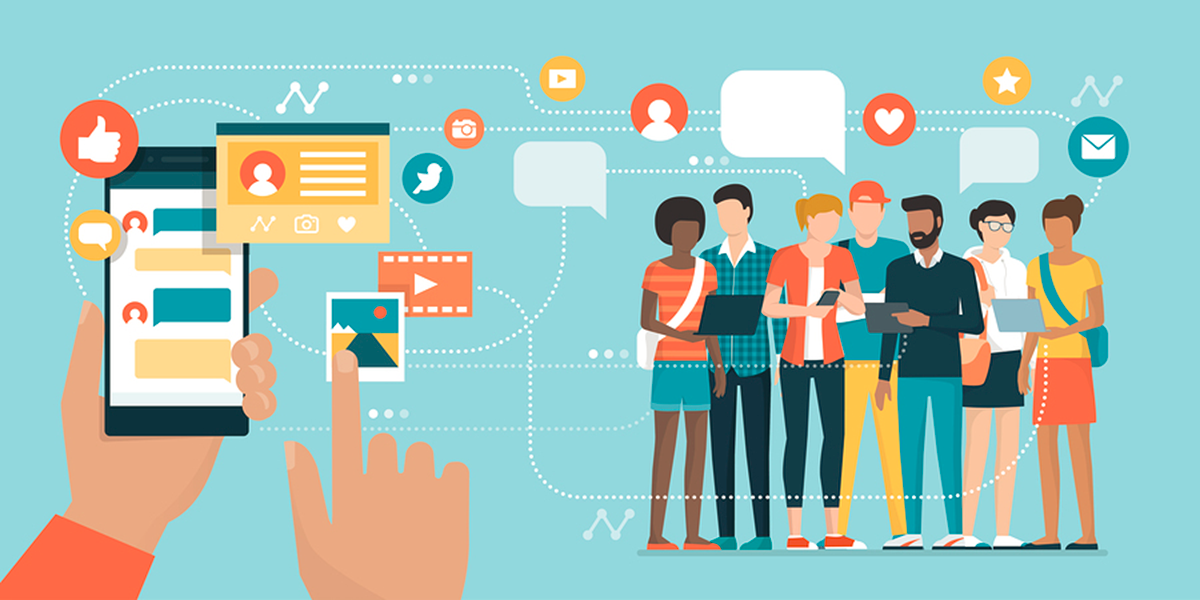
Supervised machine learning techniques for the analysis of textual and other contextual data can satisfy customers’ needs for interaction and immediacy. Driven by the current social media hype, many customers feel the need to share their opinions with companies through social media channels – via private chats, but also publicly visible through comments or posts.[36] With respect to the need for interaction, machine learning algorithms that detect response-worthy social media posts (no matter if positive or negative) can help companies identify which posts they should make a response to in order to create meaningful interactions with their customers. Similarly, also customers’ need for immediacy can be addressed, because the AI-powered detection of response-worthy social media contributions allows companies to provide an answer shortly after the contribution has been made.[37]
The development of AI applications requires a lot of technical expertise. Therefore, despite the option of purchasing complete solutions from AI-specialized firms, companies may face substantial difficulties when implementing AI applications. Irrespective of industry or company size, managers who want to create superior customer experiences should thus consider 1) what customer needs they want to satisfy during the customer journey, and 2) what capabilities are available to develop or buy AI applications that fulfill those needs.