What it looks like, how organizations benefit and customers react
Alexander Rupertus and Andreas Bayerl
The fact that prices for airline tickets or hotel rooms are different for pretty much every customer is widely accepted these days. However, dynamic pricing tactics have fought their way to previously untouched areas, such as towards non-perishable fast-moving consumer goods (FMCG), consumer durables, or even ski lift passes (e.g. in the ski resorts of St. Moritz, Sölden or Arosa). First dynamic pricing attempts by Amazon in the year 2000 were followed by heavy customer resistance and a reputational damage (Rosencrance 2000), but a recent customer survey in Germany has revealed that by now 60% of respondents accept dynamic pricing (PwC 2018). At the same time, newspapers and consumer advocates warn about companies exploiting customer data and customer trust to increase revenues (Business Insider Deutschland 2019; Jordan 2020; Kalka and Krämer 2016). How could it possibly come this far? And who benefits from dynamic pricing?
Theoretical Foundations of Dynamic Pricing
The concept of dynamic pricing has its origins in several research fields such as revenue management, yield management, or individual level price discrimination, where it has originally become of practical interest in the service industry (e.g., in the airline, travel and sporting events industry due to its adjusting supply and demand practices) and in industries that dealt with non-storable goods which had low menu costs, such as the energy business (e.g. Lauer 2018, p. 52). In contrast, for other industries like the retail industry, costs for changing prices frequently or storing unsold products were high, and thus their focus was more on improving inventory management (Elmaghraby and Keskinocak 2003, p. 1288). This has changed for two main reasons: First, technological developments, such as the rise and comfortable use of e-commerce, the quick availability and exploitation of large customer and competitor data sets, and the easy availability of addressing multiple international target markets have set the basis for companies to use dynamic pricing tactics (Bradlow et al. 2017, p. 93; Chen, Mislove, and Wilson 2016, p. 1348). Secondly, automated price setting enabled by software developments and newly introduced electronic price tags in offline stores nowadays allow for quick and cheap changes (Brynjolfsson and Smith 2000, p. 580; Grewal et al. 2011, p. S46).
How dynamic pricing can be implemented in practice

One of the most prominent dynamic pricing forms is time-based (or intertemporal) dynamic pricing (Lauer 2018, p. 58). The customer is confronted with “take-it-or-leave-it” prices, which the seller (or a selling platform) dynamically changes over time, whereas all customers are offered an equal price at the same point of time (Elmaghraby and Keskinocak 2003, p. 1288). Considering that price changes have previously occurred over a more extended period, they nowadays might change up to several times per day (Chen, Mislove, and Wilson 2016, p. 1348).
The second very prominent form of dynamic pricing is consumer-based dynamic pricing, including several special notations such as personalized-, behavior-based or targeted dynamic pricing (e.g. Priester, Robbert, and Roth 2020, p. 99). This form of dynamic pricing differentiates prices between customers for the identical product or service at the same point of time to match each customer’s maximum willingness-to-pay and to skim all consumer surplus (Choudhary et al. 2005; Garbarino and Lee 2003, p. 496).
A third and newer form of dynamic pricing is channel-based dynamic pricing, which has emerged especially through the development and use of the Internet. It is relevant for sellers that use several channels, such as an online, offline, mobile app, or indirect selling via a selling platform or a wholesaler that would like to differentiate the prices between channels to match with higher costs that occur offline (Ancarani and Shankar 2004, p. 176f; Vomberg, Lauer, and Weitkämper 2020, p. 6f).
Other emerging forms of dynamic pricing that mainly exist due to the use of the Internet are, in the field of auctions, for example, the name-your-own-price (NYOP) auction or the pay-what-you-want (PWYW) pricing model (Hinz, Hann, and Spann 2011, p. 82; Kim, Natter, and Spann 2009, p. 44f). These special forms of dynamic pricing enable the customer to discover the price mechanisms through higher transparency and to actively participate in the price-setting process (e.g. Chadran and Morwitz 2005, p. 257).
Dynamic Pricing from a Seller’s Perspective
One of the main reasons for sellers to use dynamic pricing tactics is to improve revenues and profitability by developing prices that match with the optimal willingness-to-pay of customers and the adjustment of demand and supply (e.g. Bradlow et al. 2017, p. 92; Vomberg, Lauer, and Weitkämper 2020, p. 13). Another main reason depicts increased competition in the market, especially online, as another reason to use dynamic pricing. The use of the Internet by both customers and sellers has increased the amount and availability of products, sellers, customers and exploitable data in the market (Fisher, Gallino, and Li 2018, p. 2496; Huang, Chang, and Chen 2005, p. 359f). Therefore, sellers who do not use dynamic pricing tactics and who cannot automatically adjust prices to a competitor’s price level face the risk of losing customers that switch to a lower-priced product or service of a competitor and further risk getting a poor placement on online marketplaces and price comparison websites (Chen, Mislove, and Wilson 2016). On the upside, permanently underpricing competitors can be a chance for small and unknown sellers to enter the market, gain top placement positions and build up market share, market coverage, and reputation (Choudhary et al. 2005, p. 1129).
That the use of dynamic pricing methods has the potential to increase revenues can be confirmed by the results of several studies: Findings spread between three percent (Abrate, Nicolau, and Viglia 2019, p. 231) and eleven percent revenue increase (Fisher, Gallino, and Li 2018, p. 2513) up to an average margin increase of 40% per store-keeping unit (SKU) per week (Bradlow et al. 2017, p. 92). But all studies were conducted in different industries and different conditions, which makes it difficult to give a clear statement. Still, these observations are in line with those of Homburg, Lauer and Vomberg (2019, p. 609) investigating price differences between channels, who find that an average offline price premium of two percent can be implemented, but results highly differ between product segment, customer and purchase situation.
However, frequent use of dynamic pricing might increase complexity of the online market and overall reduce margins (Brynjolfsson and Smith 2000, p. 580). Further, customers discovering the use of dynamic pricing often perceive the use of them as unfair and might react unfavorably. Empirical studies revealed lower purchase intentions, decreased trust and an increasing search behavior (Weisstein, Monroe, and Kukar-Kinney 2013) that might not outweigh a price premium fostered by dynamic pricing (Anderson and Simester 2008, p. 499).
Dynamic Pricing from a Customer’s Perspective
From a customer’s perspective, dynamic pricing is predominantly perceived as a poor practice, since it discriminates and dissatisfies individuals, keeps them with a lower consumer surplus and often requires the collection of large amounts of sensitive customer data (e.g. Martin and Murphy 2017, p. 145; Xia, Monroe, and Cox 2004, p. 7). But there is reason to believe that customers can benefit from dynamic pricing tactics, too, even though this research perspective is rarely investigated. First, the increase in competition between sellers increases, which in turn can either increase the quality differentiation of products and services, or lead to a battle of discounts and lower prices for, resulting in higher customer value and customer surplus (Choudhary et al. 2005, p. 1129). Secondly, even though time-based dynamic pricing punishes customers with higher prices during peak-demand periods (e.g., Saturday afternoon at the gas station or on a Monday morning flight to a metropolis), this practice rewards other customers in low-demand periods and might even give customers access to products or services they were not able to before (e.g. a lower-priced premium-class ticket) (Spann and Skiera 2020, p. 339). Additionally, dynamic pricing, at the example of Uber or Lyft, has the power to increase supply when customer demand and willingness-to-pay are high during peak hours. Consequently, inconvenient waiting time can be reduced to typically below five minutes (Hall, Kendrick, and Nosko 2015, p. 8; Lu, Frazier, and Kislev 2018, p. 16).
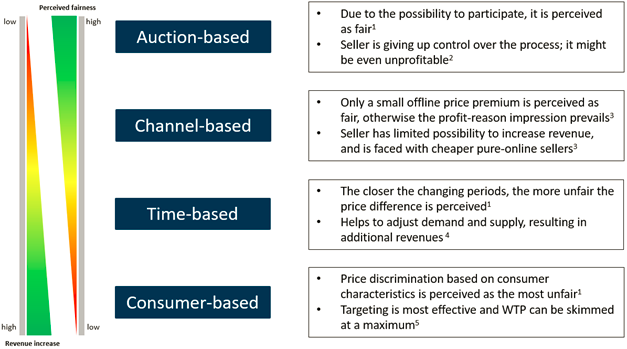
Empirical studies investigating dynamic pricing and fairness consistently prove that customers perceive these pricing tactics as unfair. More specifically, research concludes that consumer-based dynamic pricing is perceived the most unfair because it discriminates against consumers based on their individual characteristics and violates common social norms (e.g. Grewal, Hardesty, and Iyer 2004, p. 97). Therefore, even price-advantaged customers might not endorse these practices and might feel pity, embarrassment or even guilt with the price-disadvantaged customer (Xia and Kukar-Kinney 2014, p. 2374). Time-based dynamic pricing is perceived as less unfair, and auction-based dynamic pricing is the least unfair, since both do not discriminate based on individual characteristics and the latter even gives the customers the chance to participate (Haws and Bearden 2006, p. 309). Only if a price difference is caused by customer’s own participative behavior or not grounded in the intention to increase revenues or to exploit customers, but to keep up with company-external factors that are not controllable by the firm (e.g. less food supply caused by drought), customers demonstrate sympathy and do not punish the company (Vaidyanathan and Aggarwal 2003, p. 460).
Usually, dissatisfied and price-discriminated customers express their emotions in behavioral actions in form of fewer purchase intentions, increasing search behavior and loss of trust (e.g. Xia, Monroe, and Cox 2004, pp. 6–8). In the worst case, customers actively complain, spread negative WOM and directly end the relationship with the seller (e.g. Garbarino and Lee 2003, p. 509).
Discussion of Empirical Results and Strategies to Improve Fairness Perceptions
Even though a growing number of sellers have introduced dynamic pricing successfully, increased revenues, and put pressure on sellers staying with a fixed pricing scheme, the consequences of such tactics have to be critically evaluated. One stream of research findings suggests that dynamic pricing can indeed be a very valuable pricing strategy for companies and increase revenues, as well as maintaining the seller’s position in the market (e.g. Bradlow et al. 2017, p. 92). Customer-based dynamic pricing could be used to skim WTP of customers, rewarding loyal customers, or gaining switching customers (e.g., through personalized coupons sent via emails or app). Time-based dynamic pricing, instead, could be used to adjust demand and supply efficiently and strategically at the advantage of sellers. But of course, customers can also benefit from the technological possibilities, better inform themselves about prices and appropriate sellers or steer their purchase strategically (Tang and Xing 2001, p. 330).
However, the vast majority of literature streams prove that dynamic pricing upsets customers, induces negative fairness perceptions and risks to damage previously sustainable long-term relationships with customers, which might not outweigh the promised revenue increase (Lauer 2018, p. 146). Therefore, sellers should try to improve fairness perceptions by reducing unfavorable comparisons with others, which could be achieved by increasing transaction dissimilarity (Weisstein, Monroe, and Kukar-Kinney 2013, p. 512). One recently proposed possibility is to offer customers dynamic bundling, which creates customized offerings and results in dissimilar transactions (Li, Hardesty, and Craig 2018, p. 210). Another possibility to influence fairness perceptions is to frame price differences in a favorable way and mitigate negative perceptions of disadvantaged customers, e.g. in the form of a dollar-off promotion and an additional gift card (e.g. Wirtz and Kimes 2007, p. 236). The same effects can be achieved by making customers familiar of different fencing conditions (Wirtz and Kimes 2007, p. 235). Furthermore, giving customers the possibility to participate in the price-setting process, such as through negotiating, bidding, or self-selecting into certain customer segments, improves fairness perceptions significantly (e.g. Richards, Liaukonyte, and Streletskaya 2016, p. 150).

Drawing on further research, the practice of dynamic pricing to randomly gather switching customers to increase revenues can present a double-edged sword. Even though it attracts new customers in the short-term, the practice might come with disadvantages in the longer run (Feinberg, Krishna, and Zhang 2002, p. 289). As soon as customers have experienced (discriminatory) dynamic pricing, they cannot recover to previous fairness and trust levels (Lauer 2018, p. 143). In addition to that, loyal customers feel betrayed if a discount does not reward them.
As a conclusion, a simple decision regarding whether to implement dynamic pricing or not cannot be made since there are strong arguments for and against it. Each seller has to independently analyze the market situation he is confronted with, as well as his products, customers, and strategy, ponder the arguments for and against the use of dynamic pricing tactics and actively manage his pricing scheme. Future research aiming to increase generalizability of results and to investigate the influence of customer characteristics (e.g. gender, age, wealth, education) on the acceptance of dynamic pricing could offer additional guidance for managers. A new research avenue analyzing the use of electronic shelf label (ESL) and the effect of location-based personalized coupons in the offline channel would be of further interest, since an increasing number of offline retailers switch to ESL to show their prices (Lauer 2018, p. 153).